The Consistency Crapshoot
ML-assisted tools can create great results, but you have to wait and see what they are
Hi again, and thanks for subscribing to Photo AI! If you’re reading this on the web, click here to subscribe for a free or paid subscription, either of which help me as an independent journalist and photographer. As a quick reminder, I’m on Mastodon at @jeffcarlson@twit.social, co-host the podcasts PhotoActive and Photocombobulate.
First Thing
Is it fair to draw attention to something I haven’t finished yet? In this case, it’s a podcast episode. This week I learned that artist and Photoshop expert Jesús Ramirez has started a new podcast, Today’s Creator, and his first guest is Mark Heaps talking about AI: “The Truth About AI Getting ‘Creative,’ with Mark Heaps.” I’ve listened to only about a third of the episode, but need to stop and recommend it to you.
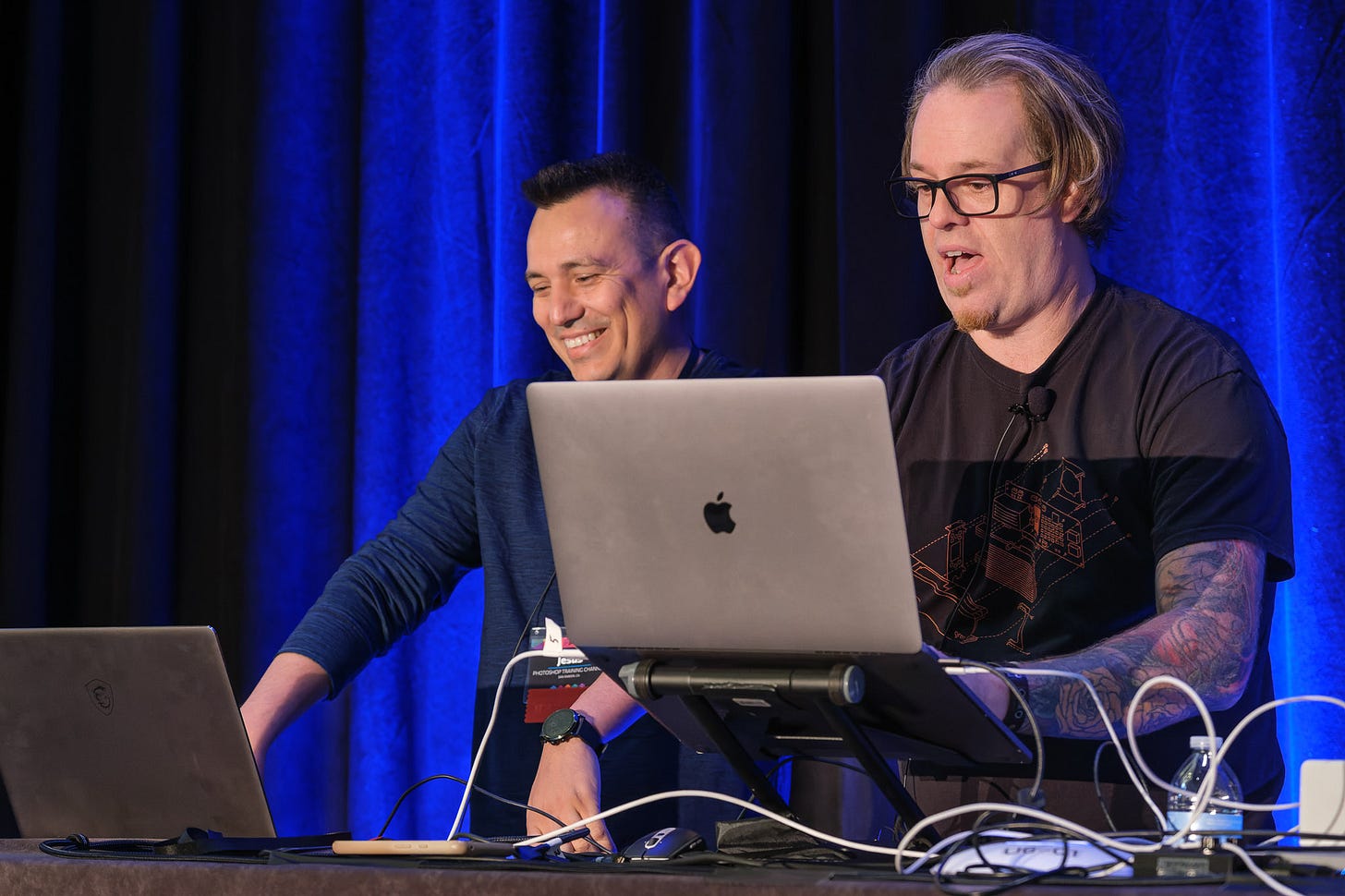
I’ve met both Jesús and Mark at CreativePro Week conferences, so I can personally vouch for their wisdom. Mark is the senior vice president of brand and creative at Groq Inc., a company building custom processors and services. He’s also a creative technologist who’s worked on imaging at Apple and Adobe and in ad agencies and… in short, he brings a lot of practical experience to the moment we’re in with AI/ML technologies affecting creative fields like photography.
The crux of what I’ve listened to so far is answering the prevailing question of whether creative folks are going to be put out of work by AI. But what I found more interesting is his discussion of “compute,” which is an aspect that doesn’t come up as much. It refers to the processing power required to create this explosion of Generative AI artwork and the cloud processing that happens with some editing features. Mark clearly and succinctly describes just how much raw data processing is needed to create the imagery we’re seeing—it’s significant. Right now everything is wide open, but we’ll no doubt see a day when GenAI could be added as a feature to Adobe Creative Cloud for an extra monthly fee, as one example. I need to finish the episode, because it’s got some other ideas in my mind swirling.
The Consistency Crapshoot
One thing Mark said that stuck with me is a reminder of the way GenAI images are created. After a text prompt is entered, the software begins with just noise, and iteratively builds an image out of it that attempts to match the parameters of the prompt.
What that also means is that the same exact prompt can produce wildly different results. He mentioned creating an image of a purple-eyed witch to accompany a story his daughter wrote. After several attempts to hit on the right combination of words that produced a good result, he had his image. But if he wanted another image of the same witch doing something else, it wasn’t possible with those prompts, because each image was generated from that amorphous window of noise. Using “purple-eyed witch flying” and “purple-eyed witch riding a skateboard” would create different-looking witches. (To get around that, you need to create a “seed” on which you can build other images with the same character.)
And this reminded me of how some ML-assisted tools in image editing currently work. You try to set as many parameters as you can, but ultimately you let it loose and hope you get a good result.
For instance, I’ve been mostly impressed with the results of Topaz Sharpen AI for sharpening images. It’s recently added the ability to detect subjects and work with faces in context. In some situations, it can reconstruct a motion-blurred face pretty well.
But I’ve also run into situations where the effect comes off as unnatural looking. For example, around the holidays I took some family photos including one of my wife and I. She looks great, but I was just enough past the focal plane that my face is slightly soft.
Running the image through Topaz Sharpen AI greatly improved my appearance in general, but my wife’s face looked waxy. I ultimately used Photoshop to composite a version with my sharpened face and her original face.
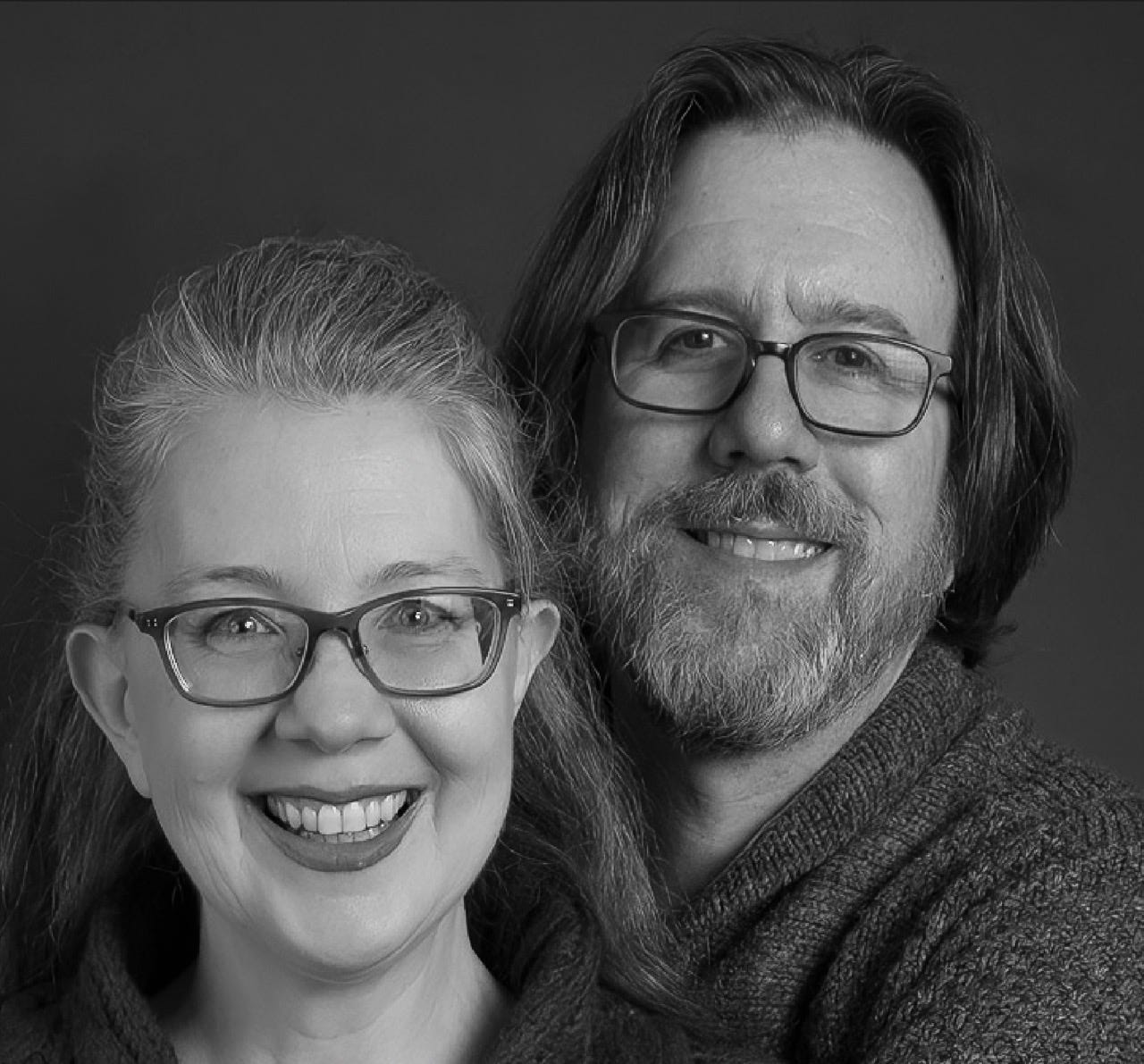
These are zoomed-in details of the full-body image, and the artifacts are more pronounced at 200% or so. It’s likely most people wouldn’t notice the effect in the full image, particularly if viewed on a phone or other device. But I notice.
As another example, I know Topaz DeNoise AI can do wonders with high-ISO noisy images, but I regularly find patches of texture where the app couldn’t figure out how to process that area.
I guess my point here is centered as much on our expectations as on what the software is delivering. We see many examples of stunning GenAI and ML-assisted images, and come away with the idea that “AI” can just magically create or fix things for us. But that’s not what’s happening. These models evaluate what’s in front of them, iterate based on previously learned data (what a “witch” looks like, for instance), evaluates that, and then iterates further. There still needs to be an artistic hand guiding the results—or rather, waiting to see what result will get spit out and then deciding how to continue.
PhotoActive 135: Metadata
In the latest episode of the PhotoActive podcast that I co-host with Kirk McElhearn, we look at metadata, including how apps like Apple Photos, Adobe Lightroom, and Excire Foto are using AI to bypass the process of manually keywording and describing images in your libraries. Listen and subscribe here: PhotoActive #135: Metadata.
Let’s Talk
Thanks again for reading and recommending Photo AI to others who would be interested. Send any questions, tips, or suggestions for what you’d like to see covered at jeff@jeffcarlson.com. Are these emails too long? Too short? Let me know.