Hello, and thanks for subscribing to Photo AI! If you’re reading this on the web, click here to subscribe for a free or paid subscription, either of which help me as an independent journalist and photographer. As a quick reminder, I’m on Mastodon at @jeffcarlson@twit.social and co-host the podcasts PhotoActive and Photocombobulate.
First Thing
Here’s something really cool. From our side of the screen, AI can be pretty magical: you click a button to make a selection, or enter a text prompt to generate artwork, or let an app pick out the better photos from a large photo shoot, and you get the results.
But AI is expensive, as in the resources needed to do that processing, and the demand for those resources keeps climbing. Systems like Adobe Sensei send requests to Creative Cloud, which distributes the computational load across numerous machines for speed and efficiency, and then zaps a result back to your computer or device. Or, the processing is done locally on your machine, which can be quick or slow depending on hardware. For example, the new M2-based MacBook Pro includes a faster 16-core Neural Engine, which will no doubt improve performance with recent macOS optimizations to Core ML targeted at Stable Diffusion.
Well, what if we could employ light to do that processing instead of vast amounts of electricity? A new IEEE article hints that’s in the works: Optical AI Could Feed Voracious Data Needs.
For example, a diffractive optical neural network is composed of a stack of layers, each possessing thousands of pixels that can diffract, or scatter, light. These diffractive features serve as the neurons in a neural network. Deep learning is used to design each layer so when input in the form of light shines on the stack, the output light encodes data from complex tasks such as image classification or image reconstruction. All this computing “does not consume power, except for the illumination light,” says study senior author Aydogan Ozcan, an optical engineer at the University of California, Los Angeles.
Add a Second Light Using Lightroom Masks
In addition to linking to interesting AI photo news and opining about various things, I want to sprinkle in some how-to topics that focus on AI and ML. These technologies are mostly about making it easier to accomplish tasks, after all, and it’s good to see them in action.
For this entry, I’m looking at masks in Lightroom, which have shifted the way I edit many of my photos. Also, I’ve been head-down working on my upcoming new book from Rocky Nook, Adobe Lightroom: A Complete Course and Compendium of Features, so Lightroom (specifically Lightroom Classic) is occupying most of my brainspace.
Here’s the situation: During a recent portrait session, I shot at a local park using a lightweight setup of a single softbox, strobe, and light stand. In this photo, it would have been nice to have another light source coming from the right to brighten the shadows on that side of my subject’s face. (I did have a reflector in my kit, but no assistant to hold it. Oh, and did I mention it had started to rain?) I like the result, but while editing I decided to add some fill light in software.
One method would be to create a new Brush mask, paint the dark portions, and increase the Shadows or Exposure values. Instead, I create a new light source, as if there was another strobe positioned out of frame, by adding a new Radial Gradient.
That lightens the shadows on his face, but now that background is too bright. This is where the AI-based masking comes in. In the Masks panel, I hold Option (Alt in Windows), which turns the Add and Subtract buttons into the Intersect button, and then choose Select Subject from the menu that appears. The mask is now limited to where the subject (the man) intersects with the Radial Gradient, knocking out the illumination in the background.
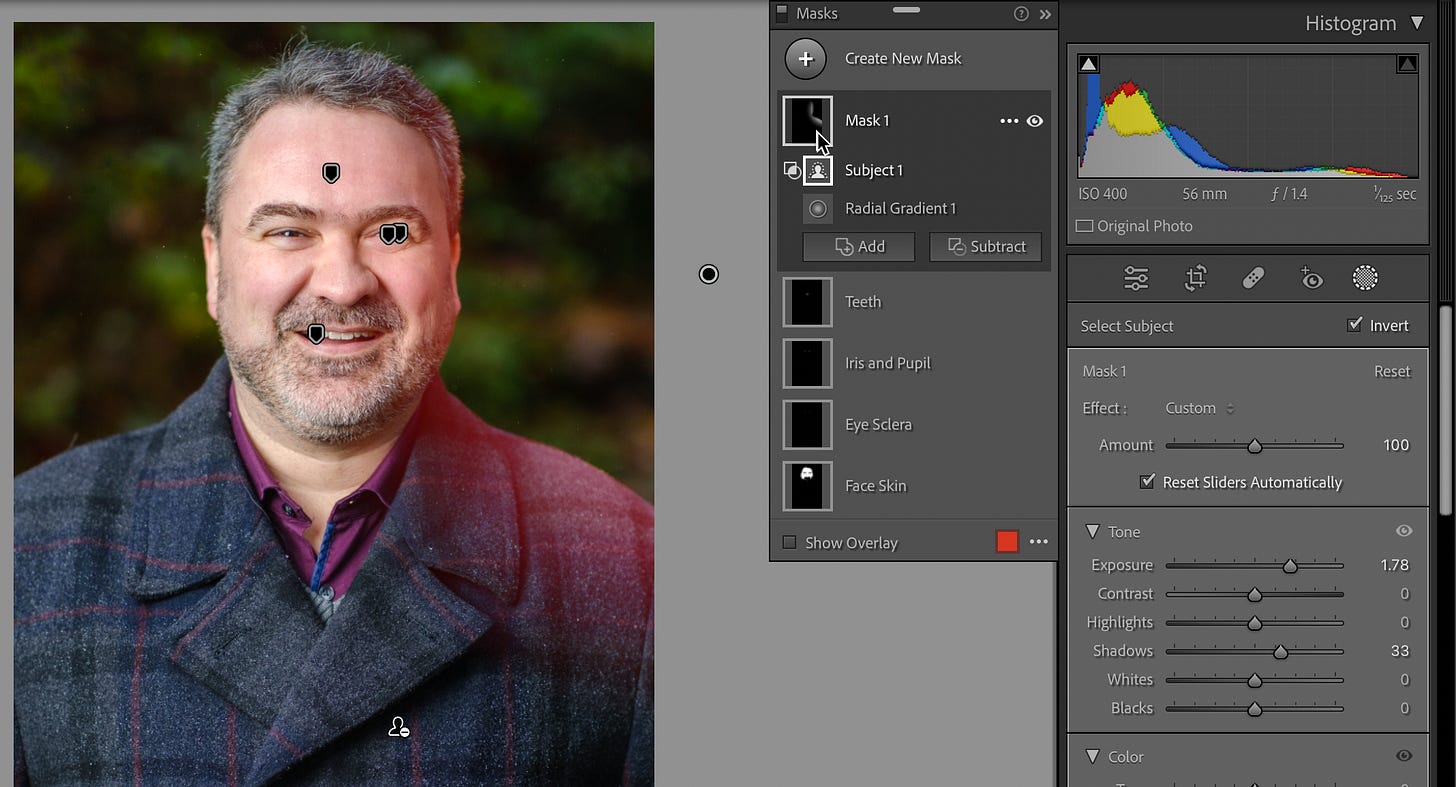
There’s still some spill on his coat, which would appear if a real light were positioned there, but I find it a little distracting. To get rid of it, I click the Subtract button, choose Brush from the menu, and paint out just that section.
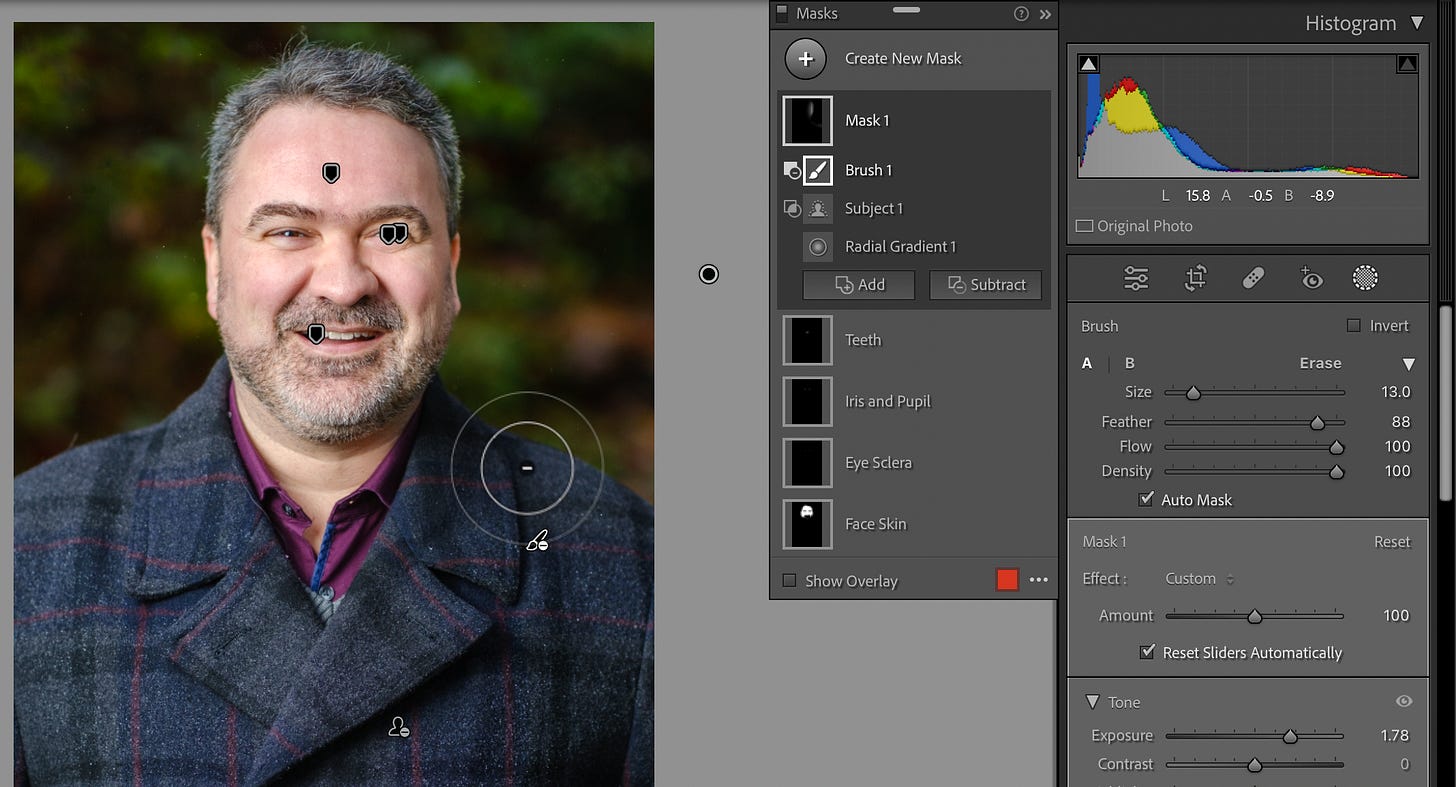
The result is some fill light that could have been a reflector or strobe. And I did it in my warm, dry office, instead of what turned out to be quite the downpour as we dashed back to our cars.
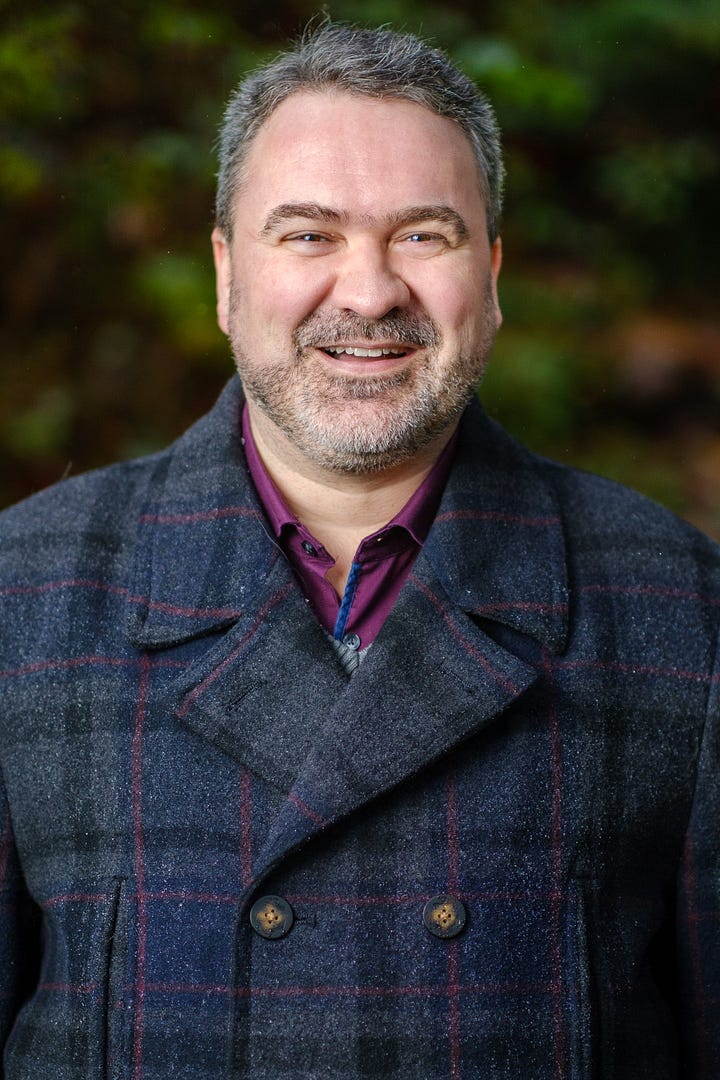
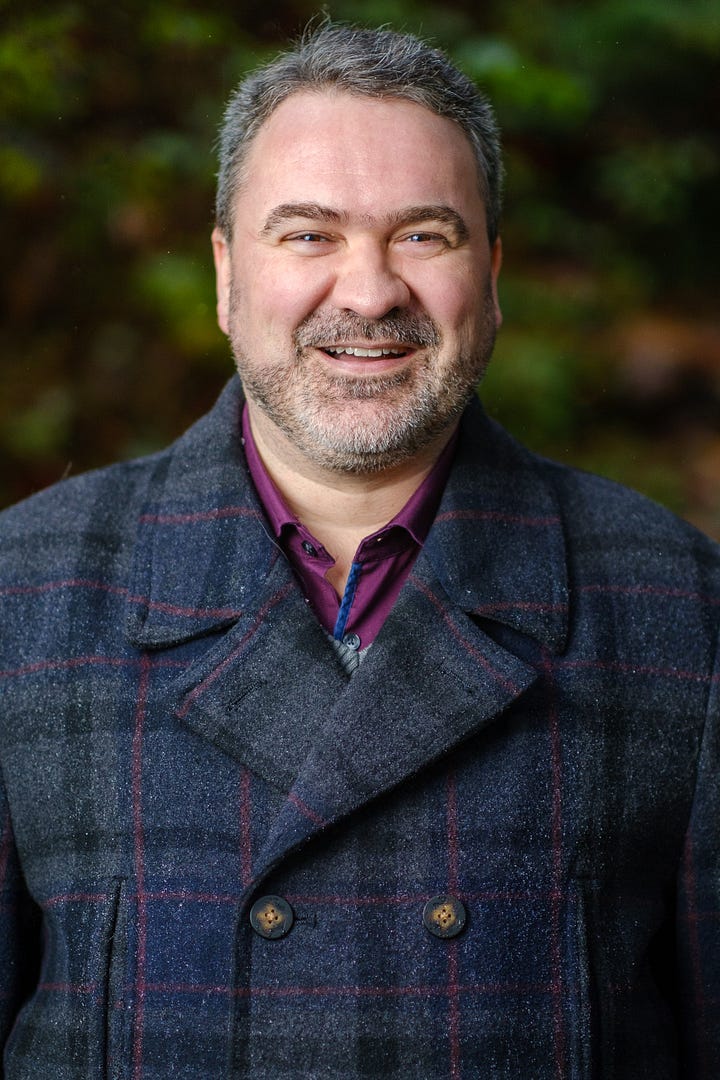
“Responsibly-Produced” Generative AI
We can’t talk about Generative AI without mentioning the ethics of how the image datasets are sourced. Shutterstock is attempting to provide a legitimate path toward creating licensable GenAI images by offering its own generator based on DALL-E technology. The part that stuck out to me in this quote is “responsibly-produced”:
"Shutterstock has developed strategic partnerships over the past two years with key industry players like OpenAI, Meta, and LG AI Research to fuel their generative AI research efforts, and we are now able to uniquely bring responsibly-produced generative AI capabilities to our own customers," says Paul Hennessy, Chief Executive Officer at Shutterstock.
Shutterstock also says the dataset is made up of “ethically created visuals,” which were sourced using Shutterstock’s own library. According to the “Shutterstock Datasets and AI-generated Content: Contributor FAQ,” the contributor TOS (terms of service) include this usage, but the company “in the coming months… will be adding an opt out function in the contributor account settings, which will allow artists to exclude their content from any future datasets or data deals if they prefer not to have their content used for training computer vision technology.” I guess if their content was already used, they’re out of luck?
What’s also interesting is that Shutterstock is going to compensate creators if their material is used in Generative AI works, via a Shutterstock Contributor Fund.
We have established a Shutterstock Contributor Fund, which will directly compensate Shutterstock contributors if their IP was used in the development of AI-generative models, like the OpenAI model, through licensing of data from Shutterstock's library. Additionally, Shutterstock will continue to compensate contributors for the future licensing of AI-generated content through the Shutterstock AI content generation tool. Earnings that resulted from the OpenAI datasets, also known as data deals, will be issued in Q4 2022.
It’s not clear how they will determine which IP is used, so I’ll be curious to see how this shakes out.
Are You Talkin’ to Me?
Lastly, a quick followup from last week’s note about Nvidia’s new Eye Contact feature for making it appear as if you’re looking at the camera during a video call. Daniel Hashimoto, a VFX artist and “Action Movie Dad” applied the technology to several movies. If you thought Anton Chigurh wasn’t creepy enough in No Country for Old Men, just wait until he’s interacting directly with you.
Let’s Talk
Thanks again for reading and recommending Photo AI to others who would be interested. Send any questions, tips, or suggestions for what you’d like to see covered at jeff@jeffcarlson.com.